ToolLLM: Facilitating Large Language Models to Master 16000+ Real-world APIs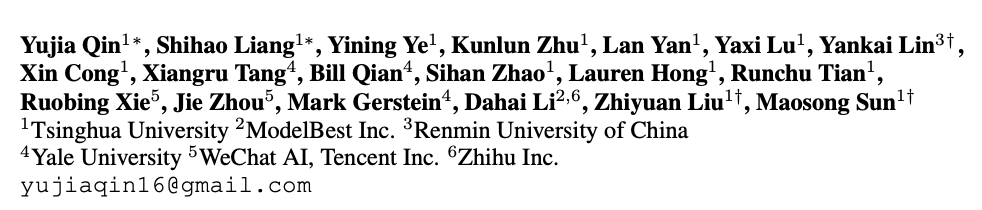
一、动机
虽然现如今大模型展现出无与伦比的表现,但是其在工具理解和使用方面依然存在不足,即根据用户的指令和意图来使用外部API。这是因为现有的指令微调任务大多数是基于语言任务,而忽略了工具的调用指令。
Although open-source LLMs, e.g., LLaMA (Touvron et al., 2023a), have achieved versatile capabilities through instruction tuning (Taori et al., 2023; Chiang et al., 2023), they still lack the sophistication in performing higher-level tasks, such as appro- priately interacting with tools (APIs) to fulfill complex human instruction.
因此,本文希望为开源的大模型探索一个可以使用工具的模型,并提出TooLLM。
目前已有一些通过指令微调来提升大模型工具使用能力的方法,但是依然存在一些局限性:
- API数量有限:缺乏一些真实场景数量丰富的API;
- 任务场景单一:大多数都是单一API工具的调用,而真实场景下可能需要多个工具协同使用;
- 规划和推理能力较差:现有研究采用 CoT(Wei 等,2023)或 ReACT(Yao 等,2022)进行模型推理,无法充分发挥 LLM 中存储的功能,因此无法处理复杂指令。此外,有些研究甚至不执行 API 来获取真实响应(Patil 等,2023;Tang 等,2023),而真实响应是后续模型规划的重要信息。
二、方法
整体的流程如下图所示:
数据构造与模型训练:
- 首先从RapidAPI网站收集大量的API,并进行采样和处理;
- 根据采样的API组合,调用ChatGPT进行指令生成,由此构造好相应的(指令,相关API)样本。这些样本可以用于训练API Retriever;
- 基于(指令,相关API),继续让ChatGPT标注这些API的组合和调用顺序,形成(指令,solution path)样本,最终形成ToolBench。这些数据用于训练ToolLLaMA模型;
推理:
- 给定一个指令,首先使用API Retriever检索所有可能的API;
- 基于这些API与RapidAPI进行多轮交互获得API的调用结果,最后获得答案。
2.1 数据构建
首先提出ToolBench,其包含了从16464个Rapid API网站上爬取处理过的API。其数量统计与其他Benchmark对比如下表所示:
TooBench的构建有三个步骤:
- API收集
- 指令构建
- Tool Path标注
(1)API收集
API主要来自于RapidAPI网站,其提供了49个粗粒度API的类别(Categories):
以及超过500个细粒度的类别(Collections)
每个tool会包含若干个API,每个tool将获取其名称、描述、URL以及所有涉及的API。对于每个API,也同时获得其名称、描述、HTTP、调用所需参数、request body信息、API的执行代码片段以及response样例等。这些元信息将有助于大模型来理解并使用API。
RapidAPI的层次结构如下图所示:
(2)指令构造
ToolBench的构造是为了后续让开源大模型能够更好地进行指令微调和工具的理解,因此,需要考虑乳腺癌两个场景:
- diversity:需要提供多样的工具使用场景,使得大模型具有工具泛化能力;
- multi-tool usage:需要符合真实场景,即一个问题可能需要多个工具协同完成。
首先对于所有的API集合中,随机采样一些API。其次设计prompt让ChatGPT理解这些API,并生成出如下两个内容:
- instruction:生成一个指令,使得这个指令可以涉及到所采样的这些API;
- relevant APIs:从被采样的API中,生成与instruction完全相关的API;
ChatGPT prompt用于提示ChatGPT的prompt包含三个部分:
- description:指令生成任务的任务描述;
- document:每个被采样的API的工具描述;
- ICL exemplar:人工编写的多个包含single-tool和multi-tool场景下的工具调用样例,并采样3个作为exemplar;
ChatGPT生成指令可以形式化为如下公式:
工具采样策略single-tool:每个tool包含若干API,所以这些API直接作为一个组合,记作single-tool instruc- tions (I1)。
multi-tool:不能随便采样组合tool,因为有一些tool之间完全没有联系。因此这里借助RapidAPI提供的分层归类结构。同属于同一个Category或者Collection的2~5个tool可以随机组合起来,且每个tool最多挑选3个API。
- 采样空间如果是同属于Category,其对应生成的instruction被称为 intra-category multi-tool instructions (I2);
- 采样空间如果同属于Collection,其对应生成的instruction被称为intra-collection multi-tool instructions (I3)
通过不同的采样策略,ChatGPT生成的指令可以具备一定的多样性。
最终生成了200k(指令,相关API)的组合样本。87413, 84815, and 25251 instances for I1, I2, and I3。
由于这些(指令,相关API)的组合样本都是由ChatGPT经过工具理解来生成的,所以这些样本可以用于训练API Retriever。
given an instruction, the API retriever recommends a set of relevant APIs, which are sent to ToolLLaMA for multi-round decision making to derive the final answer. Despite sifting through a large pool of APIs, the retriever exhibits remarkable retrieval precision, returning APIs closely aligned with the ground truth.
(3)Tool Path标注
光有instruction和relevant API还是远远不够的,因为尤其是multi-tool usage场景,这些API的调用组合和先后顺序也是至关重要的。因此,这里依然借助ChatGPT来完成API调用的组合与顺序的标注。
给定一个instruction和若干相关的API(API的名称、描述、调用方法等元信息),ChatGPT需要能够给出一个正确的动作序列。以多轮对话的形式进行。
对于每一轮,此时ChatGPT的动作需要根据上一轮的API的结果,选择下一个API。选择的API之后需要进行思考(thought),思考的内容形式为“Thought: · · · , API Name: · · · , Parameters: · · · ”。
每个动作包含两个函数:
- Finish with Final Answer:API给出的实质的结果,该动作表明API调用成功;
- Finish by Giving Up:多个API尝试调用后依然失败,或者无法完成指令所需要的内容。
基于上述的设定,提出一种基于深度优先搜索的决策树算法(DFSDT)。如下图所示:
相比宽度优先搜索,采用深度优先搜索可以较快地找到一条满足条件的路径,节省ChatGPT的调用次数。满足条件的路径即能够从instruction出发,最终完成所有API的调用。
We perform DFSDT for all the generated instructions and only retain those passed solution paths.
为此,最终获得了126486个(指令、solution path)用于训练LLaMA。
2.2 ToolEval
本文也提出了一种针对工具使用的评估体系。包含两个评价指标:
- Pass Rate:它计算在有限预算内成功完成指令的比例。该指标衡量了 LLM 指令的可执行性,可以看作是理想工具使用的基本要求;
- Win Rate:每个指令设计了两个solution path,来让ChatGPT判断哪一个正确。通常情况下,一个path是待测baseline大模型生成的tool使用路径,另一个path可以是人类编写的path,或者chatgpt所编写的ground truth。
Through rigorous testing (details in appendix A.5), we find that ToolEval demonstrates a high agreement of 87.1% in pass rate and 80.3% in win rate with human annotators. This shows that ToolEval can reflect and represent human evaluation to a large extent.
2.3 ToolLLaMA
将构建好的126486个(指令、solution path)用于训练LLaMA2-7B模型。评估的时候,根据l1、l2、l3来评估模型的工具泛化性能。
实验结果如下所示:
三、复现
假设已经有一个ToolLLaMA模型,下面通过代码来描述让大模型使用工具的过程。
给定一个指令:
[{"query": "I'm planning a surprise party for my best friend, and I want to include meaningful quotes in the decorations. Can you provide me with random love, success, and motivation quotes? It would be great to have quotes that can celebrate love, success, and inspire everyone at the party. Thank you so much for your help!","query_id": 82217}
]
执行下面代码完成工具使用:
'''
Open-domain QA Pipeline
'''
import argparse
from toolbench.inference.Downstream_tasks.rapidapi import pipeline_runnerif __name__ == "__main__":parser = argparse.ArgumentParser()parser.add_argument('--corpus_tsv_path', type=str, default="your_retrival_corpus_path/", required=False, help='')parser.add_argument('--retrieval_model_path', type=str, default="your_model_path/", required=False, help='')parser.add_argument('--retrieved_api_nums', type=int, default=5, required=False, help='')parser.add_argument('--backbone_model', type=str, default="toolllama", required=False, help='chatgpt_function or davinci or toolllama')parser.add_argument('--openai_key', type=str, default="", required=False, help='openai key for chatgpt_function or davinci model')parser.add_argument('--model_path', type=str, default="your_model_path/", required=False, help='')parser.add_argument('--tool_root_dir', type=str, default="your_tools_path/", required=True, help='')parser.add_argument("--lora", action="store_true", help="Load lora model or not.")parser.add_argument('--lora_path', type=str, default="your_lora_path if lora", required=False, help='')parser.add_argument('--max_observation_length', type=int, default=1024, required=False, help='maximum observation length')parser.add_argument('--max_source_sequence_length', type=int, default=4096, required=False, help='original maximum model sequence length')parser.add_argument('--max_sequence_length', type=int, default=8192, required=False, help='maximum model sequence length')parser.add_argument('--observ_compress_method', type=str, default="truncate", choices=["truncate", "filter", "random"], required=False, help='maximum observation length')parser.add_argument('--method', type=str, default="CoT@1", required=False, help='method for answer generation: CoT@n,Reflexion@n,BFS,DFS,UCT_vote')parser.add_argument('--input_query_file', type=str, default="", required=False, help='input path')parser.add_argument('--output_answer_file', type=str, default="",required=False, help='output path')parser.add_argument('--toolbench_key', type=str, default="",required=False, help='your toolbench key to request rapidapi service')parser.add_argument('--rapidapi_key', type=str, default="",required=False, help='your rapidapi key to request rapidapi service')parser.add_argument('--use_rapidapi_key', action="store_true", help="To use customized rapidapi service or not.")parser.add_argument('--api_customization', action="store_true", help="To use customized api or not. NOT SUPPORTED currently under open domain setting.")args = parser.parse_args()# 执行函数,由于只有指令,所以需要先设置add_retrieval=Truepipeline_runner = pipeline_runner(args, add_retrieval=True)pipeline_runner.run()
- 读取当前的指令数据,构造任务数据
def generate_task_list(self):args = self.argsquery_dir = args.input_query_file # 待执行指令所属的文件answer_dir = args.output_answer_fileif not os.path.exists(answer_dir):os.mkdir(answer_dir)method = args.method # 例如DFS_woFilter_w2,即采用DFS来寻找API call solution pathbackbone_model = self.get_backbone_model() # 例如训练好的ToolLLaMA模型white_list = get_white_list(args.tool_root_dir) # 获得一些已有的tooltask_list = []querys = json.load(open(query_dir, "r")) # 读取所有待测的指令for query_id, data_dict in enumerate(querys):# 遍历每一个待测指令if "query_id" in data_dict:query_id = data_dict["query_id"]if "api_list" in data_dict:origin_tool_names = [standardize(cont["tool_name"]) for cont in data_dict["api_list"]]tool_des = contain(origin_tool_names,white_list)if tool_des == False:continuetool_des = [[cont["standard_tool_name"], cont["description"]] for cont in tool_des]else:tool_des = None# 最终,每个指令都将对应一个任务,任务包含了推理的方法、backbone等信息task_list.append((method, backbone_model, query_id, data_dict, args, answer_dir, tool_des))return task_list
- 推理
def run(self):# 每个指令对应一个任务task_list = self.task_listrandom.seed(42)random.shuffle(task_list)print(f"total tasks: {len(task_list)}")new_task_list = []for task in task_list:out_dir_path = task[-2]query_id = task[2]output_file_path = os.path.join(out_dir_path,f"{query_id}_{self.args.method}.json")if not os.path.exists(output_file_path):new_task_list.append(task)task_list = new_task_listprint(f"undo tasks: {len(task_list)}")if self.add_retrieval:# 如果只有指令,则需要调用retriever来获取可能的APIretriever = self.get_retriever()else:retriever = Nonefor k, task in enumerate(task_list):print(f"process[{self.process_id}] doing task {k}/{len(task_list)}: real_task_id_{task[2]}")# 执行API调用推理result = self.run_single_task(*task, retriever=retriever, process_id=self.process_id)
- Tool Retriever:采用向量检索的办法,根据指令获取topK个工具。
import time
import pandas as pd
from sentence_transformers import SentenceTransformer, util
import json
import re
from toolbench.utils import standardize, standardize_category, change_name, process_retrieval_ducomentclass ToolRetriever:def __init__(self, corpus_tsv_path = "", model_path=""):self.corpus_tsv_path = corpus_tsv_pathself.model_path = model_pathself.corpus, self.corpus2tool = self.build_retrieval_corpus()self.embedder = self.build_retrieval_embedder()self.corpus_embeddings = self.build_corpus_embeddings()def build_retrieval_corpus(self):print("Building corpus...")documents_df = pd.read_csv(self.corpus_tsv_path, sep='\t')corpus, corpus2tool = process_retrieval_ducoment(documents_df)corpus_ids = list(corpus.keys())corpus = [corpus[cid] for cid in corpus_ids]return corpus, corpus2tooldef build_retrieval_embedder(self):print("Building embedder...")embedder = SentenceTransformer(self.model_path)return embedderdef build_corpus_embeddings(self):print("Building corpus embeddings with embedder...")corpus_embeddings = self.embedder.encode(self.corpus, convert_to_tensor=True)return corpus_embeddingsdef retrieving(self, query, top_k=5, excluded_tools={}):print("Retrieving...")start = time.time()query_embedding = self.embedder.encode(query, convert_to_tensor=True)hits = util.semantic_search(query_embedding, self.corpus_embeddings, top_k=10*top_k, score_function=util.cos_sim)retrieved_tools = []for rank, hit in enumerate(hits[0]):category, tool_name, api_name = self.corpus2tool[self.corpus[hit['corpus_id']]].split('\t') category = standardize_category(category)tool_name = standardize(tool_name) # standardizingapi_name = change_name(standardize(api_name)) # standardizingif category in excluded_tools:if tool_name in excluded_tools[category]:top_k += 1continuetmp_dict = {"category": category,"tool_name": tool_name,"api_name": api_name}retrieved_tools.append(tmp_dict)return retrieved_tools
调用ToolRetriever进行检索:
def retrieve_rapidapi_tools(self, query, top_k, jsons_path):retrieved_tools = self.retriever.retrieving(query, top_k=top_k)query_json = {"api_list":[]}for tool_dict in retrieved_tools:if len(query_json["api_list"]) == top_k:breakcategory = tool_dict["category"]tool_name = tool_dict["tool_name"]api_name = tool_dict["api_name"]if os.path.exists(jsons_path):if os.path.exists(os.path.join(jsons_path, category)):if os.path.exists(os.path.join(jsons_path, category, tool_name+".json")):query_json["api_list"].append({"category_name": category,"tool_name": tool_name,"api_name": api_name})return query_json
- DFS算法,调用ToolLLaMA完成每一轮的推理,并搜索一条能够执行成功的路径
pattern = r".+_w(\d+)"
re_result = re.match(pattern,method)
assert re_result != None
width = int(re_result.group(1))
with_filter = True
if "woFilter" in method:with_filter = False
# 调用DFS算法
chain = DFS_tree_search(llm=llm_forward, io_func=env,process_id=process_id, callbacks=callbacks)
# DFS算法开始结合大模型进行推理,寻找一条路径
result = chain.start(single_chain_max_step=single_chain_max_step,tree_beam_size = width,max_query_count = max_query_count,answer=1,with_filter=with_filter)
import re
from Tree.Tree import my_tree, tree_node
from Prompts.ReAct_prompts import FORMAT_INSTRUCTIONS_SYSTEM_FUNCTION, FORMAT_INSTRUCTIONS_USER_FUNCTION
from Prompts.Tree_search_prompts import DIVERSITY_PROMPT
from Algorithms.base_search import base_search_method
from copy import deepcopy
from LLM_rank.rank_candidate import sum_based_rankn, rank2_subfix
import json
import randomclass DFS_tree_search(base_search_method):def __init__(self, llm, io_func, process_id=0, callbacks=None):super(DFS_tree_search, self).__init__(llm, io_func, process_id, callbacks)"""Depth-first search. with_filter=True: Every time a child node is generated, choose the best multiple iterations to go.with_filter=False: Do as Preorder traversal."""self.io_func = io_funcself.llm = llmself.process_id = process_idself.restart()self.callbacks = callbacks if callbacks is not None else []def restart(self):self.status = 0self.terminal_node = []self.give_up_node = []self.now_expand_num = 0self.query_count = 0self.total_tokens = 0def send_agent_chain_end(self, depth, agent_block_ids, chain_block_ids):for i in range(len(self.callbacks)):callback = self.callbacks[i]callback.on_chain_end(depth=depth,block_id=chain_block_ids[i])if i < len(agent_block_ids):callback.on_agent_end(depth=depth,block_id=agent_block_ids[i])def to_json(self, answer=False, process=True):if process:json_obj = {"win": self.status == 1,"tree": self.tree.to_json_recursive(),"forward_args": self.forward_args,"compare_candidates": [],}for node in self.terminal_node:if node.pruned == False: # has answerjson_obj["compare_candidates"].append(node.get_chain_result_from_this_node(use_messages=False))else:json_obj = {}if answer:json_obj["answer_generation"] = {"valid_data": False,"query_count": self.query_count,"total_tokens": self.total_tokens,"final_answer": "","finish_type": "give_answer","function": self.io_func.functions,"chain": [],}for node in self.terminal_node:if node.pruned == False:json_obj["answer_generation"]["valid_data"] = Truejson_obj["answer_generation"]["finish_type"] = "give_answer"json_obj["answer_generation"]["final_answer"] = node.descriptionjson_obj["answer_generation"]["train_messages"] = node.get_train_messages_from_this_node()break# do not have final answer, look for give_upif json_obj["answer_generation"]["valid_data"] == False:if len(self.give_up_node) > 0:random_pos = random.randint(0, len(self.give_up_node) - 1)choose_give_up_node = self.give_up_node[random_pos]json_obj["answer_generation"]["valid_data"] = Truejson_obj["answer_generation"]["finish_type"] = "give_up"json_obj["answer_generation"]["final_answer"] = choose_give_up_node.descriptionjson_obj["answer_generation"]["train_messages"] = choose_give_up_node.get_train_messages_from_this_node()return json_objdef start(self, single_chain_max_step, tree_beam_size, max_query_count, answer=1, with_filter=True):""" single_chain_max_step: The maximum depth of the treetree_beam_size: How many children nodes for one node are generated per layeranswer = n means the Algo exits when find n "give_answer" nodesmax_query_count: the Algo exits when OpenAI-query exists this valuewith_filter: This is the difference between normal DFS(with_filter=True) and DFSDT(with_filter=False). """self.forward_args = locals()if "self" in self.forward_args.keys():self.forward_args.pop("self")self.tree = my_tree()self.tree.root.node_type = "Action Input"self.tree.root.io_state = deepcopy(self.io_func)system = FORMAT_INSTRUCTIONS_SYSTEM_FUNCTIONsystem = system.replace("{task_description}",self.io_func.task_description)self.tree.root.messages.append({"role": "system", "content": system})user = FORMAT_INSTRUCTIONS_USER_FUNCTIONuser = user.replace("{input_description}",self.io_func.input_description)self.tree.root.messages.append({"role": "user", "content": user})return self.DFS(self.tree.root, single_chain_max_step, tree_beam_size, max_query_count, answer, with_filter)def DFS(self, now_node, single_chain_max_step, tree_beam_size, max_query_count, answer, with_filter=True):"""Returns the number of grids to go back. When a child node of a node generates a final answer or give up, it should go back a few more gridsIn a sense, the larger this value is, the more diverse it is, and it is GreedySearch@n when it is enlarged to infinity."""# this two value declares the rate to go back, Algo degrades to CoT when the value=Inffinal_answer_back_length = 2prune_back_length = 2now_node.expand_num = self.now_expand_numself.now_expand_num += 1if now_node.get_depth() >= single_chain_max_step or now_node.pruned or now_node.is_terminal:if now_node.is_terminal: # final answerself.status = 1self.terminal_node.append(now_node)return final_answer_back_lengthelse:now_node.pruned = Trueif now_node.observation_code == 4:self.give_up_node.append(now_node)return prune_back_lengthelse:return 1next_tree_split_nodes = []for i in range(tree_beam_size):temp_now_node = now_node"""If a node have children now, We will prompt the model to generate different nodes than all the existing nodes"""delete_former_diversity_message = Falsediversity_message = Noneif len(temp_now_node.children) > 0:former_candidates_des = ""js_list = []for k, child in enumerate(temp_now_node.children):temp_node = childwhile not temp_node.is_terminal and temp_node.node_type != "Action Input" and len(temp_node.children) > 0:temp_node = temp_node.children[0]if temp_node.node_type == "Action Input":obj_dict = {"name": temp_node.father.description,"arguments": temp_node.description,"function_output": temp_node.observation,"mento-carlo-action-value": temp_node.compute_weight(),}js_list.append(obj_dict)if len(js_list) > 0:former_candidates_des = former_candidates_des + \f"{json.dumps(js_list,indent=2)}\n"if temp_now_node.observation != "":former_candidates_des = former_candidates_des + \f"again, your former observation: {temp_now_node.observation}\n"diverse_prompt = DIVERSITY_PROMPTdiverse_prompt = diverse_prompt.replace("{previous_candidate}", former_candidates_des)diversity_message = {"role": "user", "content": diverse_prompt}temp_now_node.messages.append(diversity_message)delete_former_diversity_message = True# on_chain_startnow_depth = temp_now_node.get_depth() // 3chain_block_ids = [callback.on_chain_start(depth=now_depth,inputs=temp_now_node.messages) for callback in self.callbacks]agent_block_ids = []self.llm.change_messages(temp_now_node.messages)# on_llm_start[callback.on_llm_start(depth=now_depth,messages=temp_now_node.messages) for callback in self.callbacks]new_message, error_code, total_tokens = self.llm.parse(self.io_func.functions, process_id=self.process_id)# on_llm_end[callback.on_llm_end(depth=now_depth,response=new_message) for callback in self.callbacks]self.query_count += 1self.total_tokens += total_tokensif self.query_count >= max_query_count: # a big return value will cause the Algo to exitreturn 100000# We need to exclude the diversity_message, because it will influence child nodesif delete_former_diversity_message:temp_now_node.messages[-1]["valid"] = False# parse nodes from OpenAI-message like CoT methodassert new_message["role"] == "assistant"if "content" in new_message.keys() and new_message["content"] != None:temp_node = tree_node()temp_node.node_type = "Thought"temp_node.description = new_message["content"]child_io_state = deepcopy(temp_now_node.io_state)child_io_state.retriever=Nonetemp_node.io_state = child_io_statetemp_node.is_terminal = child_io_state.check_success() != 0temp_node.messages = deepcopy(temp_now_node.messages)temp_node.father = temp_now_nodetemp_now_node.children.append(temp_node)temp_node.print(self.process_id)temp_now_node = temp_nodeif error_code != 0:temp_now_node.observation_code = error_codetemp_now_node.pruned = Trueif "function_call" in new_message.keys():# on_agent_actionagent_block_ids = [callback.on_agent_action(depth=now_depth,action=new_message["function_call"]["name"],action_input=new_message["function_call"]["arguments"]) for callback in self.callbacks]function_name = new_message["function_call"]["name"]temp_node = tree_node()temp_node.node_type = "Action"temp_node.description = function_namechild_io_state = deepcopy(temp_now_node.io_state)child_io_state.retriever=Nonetemp_node.io_state = child_io_statetemp_node.is_terminal = child_io_state.check_success() != 0temp_node.messages = deepcopy(temp_now_node.messages)temp_node.father = temp_now_nodetemp_now_node.children.append(temp_node)temp_node.print(self.process_id)temp_now_node = temp_nodefunction_input = new_message["function_call"]["arguments"]temp_node = tree_node()temp_node.node_type = "Action Input"temp_node.description = function_inputchild_io_state = deepcopy(temp_now_node.io_state)child_io_state.retriever=None# on_tool_start[callback.on_tool_start(depth=now_depth,tool_name=temp_now_node.description,tool_input=function_input) for callback in self.callbacks]observation, status = child_io_state.step(action_name=temp_now_node.description, action_input=function_input)temp_node.observation = observationtemp_node.observation_code = statustemp_node.io_state = child_io_statetemp_node.is_terminal = child_io_state.check_success() != 0temp_node.messages = deepcopy(temp_now_node.messages)temp_node.father = temp_now_nodetemp_now_node.children.append(temp_node)temp_node.print(self.process_id)temp_now_node = temp_node# on_tool_end[callback.on_tool_end(depth=now_depth,output=observation,status=status) for callback in self.callbacks]if status != 0:# return code defination can be seen in Downstream_tasks/rapid_apiif status == 4:temp_now_node.pruned = Trueelif status == 1: # hallucination api nameassert "function_call" in new_message.keys()new_message["function_call"]["name"] = "invalid_hallucination_function_name"elif status == 3: # final answertemp_now_node.is_terminal = Truetemp_now_node.make_finish(final_answer_back_length)temp_now_node.messages.append(new_message)if temp_now_node.node_type == "Action Input":temp_now_node.messages.append({"role": "function","name": new_message["function_call"]["name"],"content": temp_now_node.observation,})return_value = Noneif not with_filter: # DFSDTresult = self.DFS(temp_now_node, single_chain_max_step,tree_beam_size, max_query_count, answer, with_filter)if len(self.terminal_node) >= answer:return_value = 10000elif result > 1:return_value = result-1else:next_tree_split_nodes.append(temp_now_node)self.send_agent_chain_end(now_depth, agent_block_ids, chain_block_ids)if return_value is not None:return return_value# Sort the generated next_tree_split_nodes nodes when normal DFSif len(next_tree_split_nodes) > 1:# When using normal DFS, if we have many child nodes, we will refer to LLM to compare and choose the best one to expand first# remember, this operator will cost extra OpenAI calls.LLM_rank_args = {"functions": self.io_func.functions,"process_id": self.process_id,"task_description": self.io_func.task_description,"rank_func": rank2_subfix,}scores, rank_query_count, total_tokens = sum_based_rankn(self.llm, LLM_rank_args=LLM_rank_args, candidates=next_tree_split_nodes)self.query_count += rank_query_countself.total_tokens += total_tokensfor score, node in zip(scores, next_tree_split_nodes):node.prior_score = scorezip_value = list(zip(next_tree_split_nodes, range(len(next_tree_split_nodes))))zip_value.sort(key=lambda x: x[0].prior_score, reverse=True) # 先做score高的next_tree_split_nodes, filtered_order = zip(*zip_value)# if self.process_id == 0:# print(f"score={scores}, filtered order: {filtered_order}")'''Choose one to expand'''for i in range(len(next_tree_split_nodes)):result = self.DFS(next_tree_split_nodes[i], single_chain_max_step, tree_beam_size, max_query_count, answer)if len(self.terminal_node) >= answer:return 10000elif result > 1:now_node.make_finish(2)return result - 1return 1
由于ToolBench数据构造时也用到了DFS,此时调用的LLM是ChatGPT。在Evaluation推理时则是ToolLLaMA,所以ToolLLaMA推理的格式需要与OpenAI保持一致。